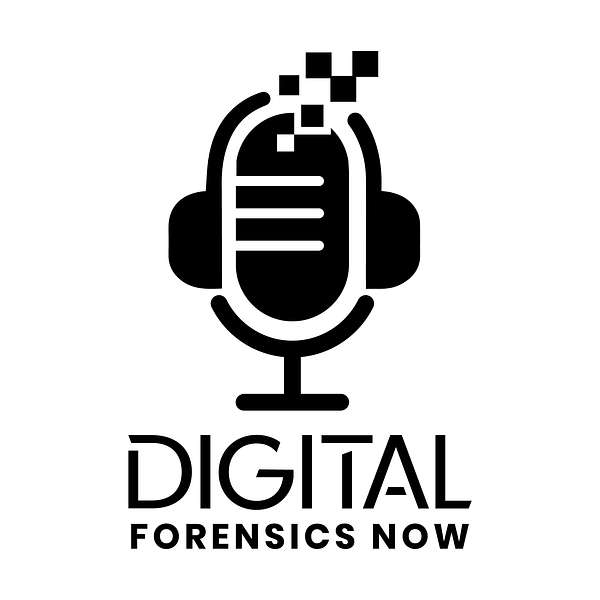
Digital Forensics Now
A podcast by digital forensics examiners for digital forensics examiners. Hear about the latest news in digital forensics and learn from researcher interviews with field memes sprinkled in.
Digital Forensics Now
The Iceberg of Digital Evidence: What AI Can't See
The boundary between tool-dependent analysis and true forensic expertise grows increasingly blurred as AI enters the digital forensics landscape. Alexis Brignoni and Heather Charpentier reunite after a month-long hiatus to sound the alarm on a concerning trend: the integration of generative AI into forensic tools without adequate safeguards for verification and validation.
Drawing from Stacey Eldridge's firsthand experience, they reveal how AI outputs can be dangerously inconsistent, potentially creating false positives (or missing critical evidence) while providing no reduction in examination time if proper verification procedures are followed. This presents investigators with a troubling choice: trust AI results and save time but risk severe legal and professional consequences, or verify everything and negate the promised efficiency benefits. The hosts warn that as AI becomes ubiquitous in forensic tools, it dramatically expands the attack surface for challenging evidence in court—especially when there's no traceability of AI prompts, responses, or error rates.
Beyond the AI discussion, the episode delivers practical insights for investigators, including an in-depth look at the Android gallery trash functionality. When users delete photos, these files remain in a dedicated trash directory for 30 days with their original paths and deletion timestamps fully preserved in the local DB database—a forensic goldmine for cases where suspects attempt to eliminate evidence shortly before investigators arrive. Other highlights include recent updates to the Unfurl tool for URL analysis, Parse SMS for recovering edited and unsent iOS messages, and Josh Hickman's research on Apple CarPlay forensics.
Whether you're investigating distracted driving cases, analyzing group calls on iOS, or simply trying to navigate the increasingly complex digital evidence landscape, this episode offers both cautionary wisdom and practical techniques to enhance your forensic capabilities. Join the conversation as we explore what it truly means to be a digital forensic expert in an age of increasing automation.
Ready to strengthen your digital investigation skills? Subscribe now for more insights from the front lines of digital forensics.
Notes:
Magnet Virtual Summit Presentations
https://www.magnetforensics.com/magnet-virtual-summit-2025-replays/
https://www.stark4n6.com/2025/03/magnet-virtual-summit-2025-ctf-android.html
parse_smsdb
https://www.linkedin.com/posts/alberthui_ios-16-allows-for-imessagesmsmmsrcs-message-activity-7279586088988413952-xHWl
https://github.com/h4x0r/parse_sms.db/tree/main
Are you a DF/IR Expert Witness or Just a Useful Pawn?
https://www.linkedin.com/posts/dfir-training_a-pawn-moves-where-its-told-a-dfir-expert-activity-7292981112463572992-c3wd/
Unfurl
https://dfir.blog/unfurl-parses-obfuscated-ip-addresses/
https://github.com/obsidianforensics/unfurl
AI to Summarize Chat Logs and Audio from Seized Mobile Phones
https://www.404media.co/cellebrite-is-using-ai-to-summarize-chat-logs-and-audio-from-seized-mobile-phones/
Ridin' With Apple CarPlay 2
https://thebinaryhick.blog/2025/02/19/ridin-with-apple-carplay-2/
Hello Who is on the Line?
https://metadataperspective.com/2025/02/05/hello-who-is-on-the-line/
Welcome to the Digital Forensics Now podcast. Today is Thursday, March the 6th 2025. My name is Alexis Brignoni, aka Briggs, and I'm accompanied by my co-host, my dear friend and my empathic dear Heather Charpentier. The music is higher up and I forgot the author of the song, Shane Ivers. Shane Ivers, thank you and can be found at silvermansoundcom. There we go, I got it.
Speaker 1:So, when I had the script right and the screen that I have my second screen. The resolution is not the best, it's an old, cheapo screen, so I kind of tried to send it down and not online and I deleted the line. So oh geez anyways, we made it.
Speaker 2:We made it here, it's because we, it's because we took a month off. You forgot who our music was by that?
Speaker 1:that is true. That is true, I'm I'm old and senile well, yeah you don't have to, you don't have to comment on that.
Speaker 2:You can just let it go I'm just gonna go yeah, let it go, let it go. I'm just going to go yeah, let it go, let it go, anyways hi everybody.
Speaker 1:We're so happy that you're here, the folks that are coming in live, kevin, the man with the master plan, the forensic wizard, johan is here again, our right-hand person, hi, johan. Laurie is also here, and Christian doing all the good stuff that he does with YouFade and his participation in the community and social media. So hi to everybody. So, heather, so what's going on? What's going on? We've been in a little bit of hiatus, so what happened with the hiatus.
Speaker 2:There's been so much stuff going on that I've felt so busy and I know that you have too so we kind of just haven't been able to have the podcast the last few weeks, so we took the month of February off. Um.
Speaker 1:I mean kind of, I mean off of the podcast, but not all the other stuff. We have to do.
Speaker 2:It's been crazy, not off from everything else, but um. I mean, I, I've been just like really busy at work. We We've been doing some job fairs at the state police, and so I have to share a picture from the job fair yesterday at UAlbany in.
Speaker 2:Albany. So this is a couple of our digital forensic or computer forensic analysts at the lab and they came over and helped myself and my friend Kevin with the job fair. I made them pose for the picture for all the social media. But tons of great students over there asking all kinds of great questions, so I'll take some of them and then throw some your way for the. Fbi as well.
Speaker 1:I don't think I can be picking anybody at this point.
Speaker 2:Yeah, all right, maybe I'll just keep them all, because there were some really good people stopping by the point. Yeah, all right, maybe I'll just keep them all, cause there were some really good people stopping by the booth.
Speaker 1:Yeah, that's, that's the for for now. Yes, yeah, yeah, no. Pretty cool picture. You see there a little bit of a Friday box there. Yeah, phone in and deal with it without signals, coming in, it'll you fit touch and whatnot.
Speaker 2:So pretty cool yeah we had the New York State Police swag too. We were handing out to all the students. So Kevin Pagano says which Kevin was it. I'm sorry. So I've got to tell the story because it's funny. I don't even think. I've told you so the other day. Kevin sends me a screenshot of his LinkedIn and my family is stalking him Literally the. Linkedin says. My mother looked at his LinkedIn, my sister looked at his LinkedIn and he's like the charpentiers are stalking me and so I write to them.
Speaker 2:I'm like what are you doing? Why are you guys viewing Kevin's page? And they're like well, we know you work with a couple of Kevins and we didn't know if that was one of the kevins. So we clicked on it and so I had to explain to my family. No, that's alex's kevin, and then I have my two kevins and I'd like to acquire a few more kevins.
Speaker 1:So yeah, I think, I think that I think actually is, I'm kevin's alex, that's how it actually works.
Speaker 2:Okay, uh, but there's, there's my sister in the chat. She says so many Kevins.
Speaker 1:It's Kevins all the way down. That's how it works.
Speaker 2:It is.
Speaker 1:That's how the world runs.
Speaker 2:But I had to accuse my family of stalking over the weekend.
Speaker 1:Well, you know that investigative streak goes, you know it's a family thing, you know.
Speaker 2:Yeah, definitely, definitely. Lori wants a kevin too.
Speaker 1:I'll share my kevins with anybody who wants well, um, I want to say real quick like d, for dan is here, jesse's also here, jeremy's hanging out in the chat, so it's always so good to see you all here yeah um, so yeah, so uh so what else I? Know. No, no, before something else happened that people need to know what happened, tell us what happened.
Speaker 2:So I'm leaving work to go to a meeting and I'm pulling around the campus where I work and I'm on a one-way street and there is a wrong-way driver coming down the one-way street. I'm on and she just smacks me, so I'm dealing with car damage this week as well, so you were going the wrong way.
Speaker 1:Is that what you're saying?
Speaker 2:No, but she did kindly tell the insurance company that it was a two-way street and that I sideswiped her.
Speaker 1:You know it's sad, but not unexpected. That's how people operate sometimes in this world. A lot of people operate that way.
Speaker 2:Yeah, so one of the podcasts was postponed due to me having to go take care of the image to my vehicle.
Speaker 1:Yeah, I know, I know. But you know, I mean, the good thing is that right after you got hit, right, the lady goes, you know, running away, oh yeah, I forgot about that part.
Speaker 2:She left the scene of the accident. Yeah, she didn't see the crime she left the scene and luckily there was a local PD officer up ahead that saw her going the wrong way on this road, pulled her over and questioned her about the fresh damage on her car and her response was yeah, I just hit something.
Speaker 1:Something. I mean, I had no idea what it was. That was me, the humongous car that I crashed, and now I'm fleeing the scene of the crime.
Speaker 2:I don't know how that happened. Can you come back? I need your insurance information, please, so yeah, Lori said thanks. Thank goodness you weren't injured.
Speaker 1:I wasn't injured, and neither was she, so that's really all that matters yeah, although the crash looks worse, I mean looks like kind of bad, you know yeah, I should have put the picture up. I don't have it right on me, but it's okay you know, nobody wants to see your ferrari all crashed up yes, my ferrari, my hunt, my hyundai tucson I'm not super fancy well, I mean, and you know that's, that's, it is fancy, don't be plain dumb.
Speaker 1:Also, I want to echo Lori we're happy that you're okay. That's the first thing I ask you. Are you okay? Yeah? I know you're hardheaded, but not that hardheaded, I'm making sure you're okay.
Speaker 2:My sister is chiming in. Come on, digital person, get a car cam.
Speaker 1:Well, she's calling you out now.
Speaker 2:Tell, I'm not an uber driver, sorry I'm not an uber driver and I actually do have a car cam in the box in my back room that I'm waiting for her to install for me yeah right, look, shannon is saying that now it's time to buy the ferrari. See, yeah right silver linings I'll trade her in Well on my end. Enough about me, what have you been up?
Speaker 1:to. Yeah, on my end it's. You know I don't got too much to share it's. As people might know, the federal government is going through a lot of changes, a lot of fast, really fast, and we're all having to adjust. You know it's, I don't know, I just just okay, look folks, just just read the news and you'll know what's happening and we're and we're dealing with it. So, uh, hopefully, you know, things will pan out uh positive, positively for everybody, for folks in federal government and for the nation, and we hope, always hope, for the best. That's all I have to say on that.
Speaker 2:Agree, agree completely.
Speaker 1:All right, so what do we have? So let's get into the meat of the situation here. What's happening?
Speaker 2:Yeah, I mean we have all kinds of topics because we've been tabling them for weeks. So, yeah, they have accumulated. I didn't know what to pick out of our group here, but so one of the things that I wanted to mention is the Magnet Virtual Summit that took place a couple of weeks ago last week, week before and they now have their presentations from the virtual summit up on their website to watch the recordings. So if you weren't able to attend while that was going on, they're now up and available. I just kind of wanted to highlight a couple of the segments that were on the MAGIC the MAGIC Magnet Virtual Summit presentations. So my boss, my lieutenant, actually did one of the presentations and it was titled to cloud or not to Cloud that is the question and he highlighted how digital forensic labs struggle with the decision of whether or not to take their operations to the cloud or remain on premises. So check out his presentation. He did a great job.
Speaker 1:Who was that your boss?
Speaker 2:My boss, my lieutenant Brian Salmon.
Speaker 1:Fantastic job, the best presentation at that event, right. So we highly endorse that to make sure that the boss understands that everybody needs to go see that. So thank you.
Speaker 2:You did your own presentation, but my boss's was the best presentation. Oh, absolutely.
Speaker 1:I even agree. I agree with that, so let's make sure that he knows that that's the case.
Speaker 2:There we go. Another one that I have not watched yet and I cannot wait to watch it, I just need to find the time is Kim Bradley, from Hexordia. She did a segment on the cyst diagnose logs. I really want to learn a lot more about the cyst diagnose logs and the different artifacts that you can obtain out of out of those logs, and I can't wait to watch her segment. Um, and then, alex, you did a segment in spanish about the role of the analyst beyond the use of digital forensic tools.
Speaker 1:So, um, yeah, so I've been doing the minor virtual summit, but the spanish version, I want to say for the last three or four years, maybe four, I think four, but don't quote me on it.
Speaker 1:Yeah, it's always a good time. There's a lot of the Utah functions community in Spanish is also large, but there's not enough content. I wish I had more time to create more content in Spanish. It's just time instead of premium, obviously, but it was really good.
Speaker 1:So pretty much it was uh, um, the topic was, uh, bottom pusher experts. You know, kind of in quotations. Right, we don't want to be bottom pusher experts, we want to be just plain experts, and in spanish, the, the qualifier goes at the end, right, so like expert, bottom pushers, right, um, so we don't want that last name of being the bottom pusher. And and what does that take, um, to not be a bottom pusher? And what does that take to not be a bottom pusher, even though we do have to press buttons? Don't get me wrong. Right, there's buttons that have to be pressed, and it will be the same buttons the bottom pusher presses. So there's no debate on that. But there's more behind an expert, when you do expert work, and I think that's something that is going to be even brought more to the forefront with the whole putting AI in all the data forensics tools, and that's something that we're going to discuss a little bit later down the road, I mean down the road, down the episode road.
Speaker 2:Yeah, we'll do it today.
Speaker 1:Exactly this episode that we're on right now, correct?
Speaker 2:Right. So yeah, go check out the Magnet Virtual Summit presentation. And then Christian was just in the comments too. I mentioned the SysDiagnose presentation. They used his tool, Ufade, which we featured on the podcast quite a few times, and it does an amazing job with the SysDiagnose logs.
Speaker 1:Yeah, and with the logical extraction. So that's pretty good, and people should check Christian's Ufade tool, so check it out.
Speaker 2:Definitely. Another tool that I saw on LinkedIn more recently is called Parse SMS. So this tool, starting in iOS 16, imessages you could edit them, you could unsend them. You could edit them, you could unsend them and, as of today, the author of this tool is mentioning that a lot of forensic platforms don't really display that data intuitively, so that you are missing in the SMS database. It will show you unsent messages. If there are any unsent messages in that database, it will show you edited messages and it shows you the original message and then a date that the message was edited and it will then show you what the message was edited to. Um, I have a couple of screenshots.
Speaker 1:I'm gonna just as you bring, as you bring them up, it's folks that are more like brand born, brand new into the field. Um, some of these messages, depending on the database and the service, will get populated in sequential order. So you know message, know message one ID one ID two ID three sequential order. So if you delete some let's say you deleted messages three to five there'll be that gap there that the tool will be able to highlight for you, and so that's the reason for those gap, why the gap identification is important.
Speaker 2:You can see here, in the output of that tool, exactly what Alex is talking about. We have an area here where there's a row gap, there's one row missing, and this tool will point out the rows that are missing. Uh, if you have unsent messages, there'll be a little flag next to the message or next to the um date and time that says the message was unsent. The message is no longer there when a message is unsent in the SMS database, but but there is a little flag. And then let me just pull up the other screenshot I have. I did another screenshot here. That first screenshot was from the author of the tool, but this screenshot will highlight an edited message. So you'll see, row 177 is an iMessage sent on December 18, 2022 at 1435, saying hey, hey, it's me. And then at 1436, so like one second later it was edited to hey, hey, it's me, regina.
Speaker 2:Nice, very nice, so it has some nice output and it may give you a better idea of what actually happened with those messages. I know when the edit message very first came out, some of the forensic tools were showing the original message as deleted in the parse data and then the new message as intact, which I mean I don't necessarily agree with the whole deleted, it was just edited, it was changed. So going into that database and being able to find the date edited on those messages and realize, oh, the message wasn't actually deleted, it was just edited to this.
Speaker 1:The most problematic word in data forensics is the word deleted. Yes, it is the second most problematic word is unallocated, because people will take those two words to mean either the same thing or totally different things, or things that are just like bonkers, right Right. So we're not going to get into that. But whenever you use the words deleted and unallocated, you got to make sure you define the term to the person that's hearing you, so make sure that we all understand what we're talking about.
Speaker 2:Yeah, be careful.
Speaker 1:Extremely careful.
Speaker 2:Yes, so I'll put the links for that up in the show notes. Afterwards. There's a LinkedIn post from Albert Hoy, who is the person who has the parse SMS tool, and then I'll put the link to his GitHub so everybody can download that and try it out.
Speaker 1:Absolutely yeah. I love it when folks and try it out, absolutely. Yeah, I love it when folks put those tools out.
Speaker 2:Yeah, oh, me too. I love trying them out. I have to go create test data, though. I realize I don't have any deleted messages on my phone today, so I used some old data that I had. I knew I had deleted messages.
Speaker 1:Well, yeah, there's some test data you need to create, don't forget you know. Yeah, test data you need to create, don't forget you know. Yeah, oh, I have the android almost ready for you. Yeah, it's for me. Oh, actually, I didn't tell, I didn't tell the folks for us. Well, for me and for us. So yeah, so, um, let me tell the folks real quick. I'll be going to to amsterdam at the end of the month to participate in the dx excel conference largest conference conference in the netherlands where I'll be giving the keynote and also teaching a couple of sections on the leaps and how to use them and how they work and stuff like that. So that's going to be our trial run no, I mean not trial run, I mean it's going to be a run for there and then we're going to take that content also and use it at Techno, where we've been selected you know, heather and myself to teach the leaps up at Techno, which I'm really excited. Never been to Techno, always wanted to go, so it's fun.
Speaker 2:Yeah, it'll be my first time going to Techno, so really excited about it. Yeah, anybody that's going to Techno. So, with the, the class we're doing on the leaps is a hands-on lab, and any hands-on lab at Techno you have to sign up for ahead of time. So if you're going to techno and you want to join us, make sure you go on to the site and, uh, register for that ahead of time. It will probably fill up because I think there's like 30, 30 seats, um, so come, come and join us, it'll be a good time absolutely have have had to give her her signature.
Speaker 1:You know famous.
Speaker 2:Yeah, right, um. So an article on LinkedIn that I saw within the last month is another great article from Brett Shavers. Um, I love his articles. They always hit home and it's always something that's personally happening, um, in my life, at work, whether it be, uh, in the forensic world, and I'm sure it's happening in many other people's lives.
Speaker 2:But this article is entitled Are you a DFIR Expert Witness or Just a Useful Pawn? So Brett explores the role of digital forensic and incident response professionals serving as expert witnesses in legal proceedings. He emphasizes the importance of integrity and objectivity, cautioning experts against being manipulated into serving as mere tools for one side. He advises DFIR professionals to maintain independence, thoroughly validate their findings and be prepared for rigorous cross-examination to uphold the credibility of their testimony. So a couple of my favorite quotes from this article. I'll let everybody read it, but as soon as you start looking for ways to confirm a preferred narrative instead of uncovering the truth, you're not just failing, you're corrupting the field. And my other favorite quote from it is the only thing that makes forensic work bulletproof is process. It's not your reputation, your years of experience or how well you can testify under pressure. If your methods aren't solid, you're a liability. And if your findings don't hold up, you deserve what happens in cross-examination.
Speaker 1:Oh, absolutely.
Speaker 2:I love, love this article.
Speaker 1:I find it interesting, because when let's let's be honest with ourselves here when we read something like that, what's the first thing that comes to mind? What's the first thing that comes to your mind?
Speaker 2:The first thing that comes to my mind.
Speaker 1:People shouldn't be doing this. What people are these? Oh be honest with yourself are us the first thing that comes to most people. If you're in law enforcement, you're thinking the defense, oh the defense, the defense, they're higher guns.
Speaker 2:Let's be I got you all right.
Speaker 1:Is it true or not? Yeah I mean the first thing you think is well, defense. Of course they're higher guns. You know they hire to say whatever right right, right um. That's true, that's true we immediately, we gravitate them. Uh, no, actually, when you're pointing at them, there's all these other fingers pointing at you.
Speaker 2:Um yeah, I think I was going toward the pointing at you, because I had just read the article again and I'm like oh, it's so easy to be not not to be talked into. But for those attempts to be made that you're being talked into, I need the data, I need the evidence to say this and I need you to say this to prove that. And we have to be very careful with that and make sure that we're not just conceding to that.
Speaker 1:Well, I mean, and to your point right, when we think this applies to others and we don't put ourselves first, that's a bias. And I people say you shouldn't have biases, and I mean it's a personal thing. I believe we'll always have biases. The question is, how do we manage those? Right? Do we work based on beliefs or on principles, right? And if the group and this applies, look, this needs to apply first to us on the law enforcement side. And this applies Look, this needs to apply first to us on the law enforcement side If the investigators, the prosecutor, your supervisor, the sergeant, the captain all think and believe this guy is guilty, well, I cannot operate on their belief, even if I believe it too.
Speaker 1:I cannot. I get to operate on principle and the principle will guide me. The bias will be there, but the principle will make sure to check that and make it a non-issue there. And it's us. It's us, those quotes, it's ourselves Just hitting that button, getting that output or cherry-picking.
Speaker 1:Because, let's be honest, if you have enough data, you can cherry-pick the data and make the data say whatever you want to say. And that's just a reality of any field where you have enough data. And we don't want to be in that situation, either because we're influenced by a personal bias of our belief and we shouldn't have beliefs, we should have principles or because an external entity you feel that that's that a group pressure to conform to the prevailing theory of the case and that requires that. And again, for this year, I have three main things I'm really focusing on is property, which is the more character as you, as an individual, make sure you do your due diligence, you do all the work that you need to do and not cut those corners and have proper attention to detail. When you have those three things, a lot of this, issues of maybe becoming a pawn, either by others or willfully on your own, it's not going to happen to you. Poverty, attention to detail and due diligence, those three things.
Speaker 2:I think one of the biggest pushes that I get and I know other examiners get in my office is when you have a prosecutor and they want you to say this is his phone, this is his phone. Well, we can't tell whose phone it is. We can tell you the user accounts that are in it. We can tell you what messages were being sent, If messages were being sent to a specific phone number. Um, we may find a resume saved in the documents for the person, we may find the driver's license photo in the images section, but I can't tell you that this is that defendant's device. I didn't see him with it in his hand. And then I always use the example. I have test devices and my test devices are Sheldon Cooper and Amy Farrah Fowler and if you seize them from me, nobody can go testify that those are Amy Farrah Fowler and Sheldon Cooper's devices. So definitely don't be a useful pawn.
Speaker 1:Yeah, I hate the. Can you say this game I don't like it? I mean, yeah, you got the evidence, you got my report. If you want to make some conclusion based on those, that's fine. My testimony will show. It is what it is. But this whole, look, if I could say, was his phone, guess what? Guess what. That would be already on the report.
Speaker 2:It would be on the report If it's not on the report it's because because I can't say that.
Speaker 1:I mean, come on, I mean you have the report, read it, understand it, and and that's where we go. I mean I'm not going to go out of the report because that's what it is. You know, absolutely. And and a forensic wizard says they never read the report. Look, I have so many memes about that. It's like I give you the report. Two weeks ahead we're gonna have a meeting about the report and I'm reading the report. I mean I, I I'm gonna be like, I mean let me leave it like that, right? Um, people should read the report. Let's just leave it like I'm gonna say something I shouldn't be be saying. Let's carry on.
Speaker 2:Well, I am going to say you get to the table and then you get asked the question what do we got?
Speaker 1:Page three. Let's read page three together. Let's read it together.
Speaker 2:Definitely Check out Brett's article, though, and all of his articles. He posts them on his LinkedIn. If you don't follow him on LinkedIn, you have to. He's got some great stuff.
Speaker 1:Absolutely.
Speaker 2:Brett is the best. So during our hiatus a new version of Unfurl was released. We've talked about Unfurl on the podcast before. I've shown a demo of how you can take a TikTok URL and you can put it into the unfurl tool and it'll show you timestamps for that TikTok and kind of just break that URL down into a user account, into the timestamp, into other information related to that URL. Well, the new version adds parsing of encoded and obfuscated IP addresses. It resolves blue ski handles to their identifiers and looking up their creation timestamps. And it's blue sky.
Speaker 1:I know I'm like. What service is that I never heard?
Speaker 2:about it.
Speaker 1:Is it like a beer that comes from the sky? I don't know what that is Like a blue ski? I don't know, I don't know.
Speaker 2:Blue ski annoys Alex, so I have to say it every so often, and I haven't been able to say it for a month.
Speaker 1:Oh, no, I mean, look, it doesn't annoy me, you're the one saying it, go ahead.
Speaker 2:Oh boy. So let me I'm going to put we tried, we tried out the blue sky in the new unfurl here.
Speaker 1:So as you're zooming in. Laurie mentions correctly that the unfurl is a tool developed by Ryan Benson, great examiner. He works for, she works for Google and he keeps it up. I like adding the, the, the blue sky, or like how that says brewskis or whatever as the parsing for those URLs, which is pretty neat. So show us what. What does it entail?
Speaker 2:So I use the Digital Forensics Now podcast Blue Sky page and it breaks down the profile, gives you the profile name, gives you the handle, but the thing that's new, that's added here not new, but it looks up the creation timestamp so it's showing here. I created this Blue Sky account on November 13th 2024. And that can definitely be some beneficial information for your criminal investigations. For sure, when something was created, we talked about those TikToks before. If there's an incriminating TikTok that is put up onto the TikTok platform, you can take that URL, put it into this tool and you can find out when that incriminating TikTok was created.
Speaker 1:So yeah, yeah, and this technique is really useful. I have some carved out URLs in some cases and then you can really look into the timestamps of whatever the search was within that url and and get some context. Because if you get the url outside of the database that keeps track of it in the browser, you might not have that timestamp, but if it's a search, a google search, uh, url, you might find the timestamps inside of it and encode it in a way that it's not obvious to you as a human reader. So Unfurl does that for you, does Blue Sky, does a whole bunch of stuff. So keep that in your toolbox and you might find it to be really useful when you least expect it to be.
Speaker 2:Yeah, actually too. So we recently got a new investigator in the lab and he, prior to becoming investigator, didn't have a ton of digital forensics experience, but he watched the podcast and he actually used the the episode of the podcast with the Tik TOK creation date that we showed unfurl in one of his investigations, when he was out on the road and was able to make the arrest, he said.
Speaker 1:Oh, wow, yeah, Awesome.
Speaker 2:I know he got a gold star. He's brand new. And then he used the podcast, yeah.
Speaker 1:No that that, that that makes doing the podcast, the podcast all the more worthwhile, you know exactly, Exactly.
Speaker 2:I asked him if he told that story in his interview, because I mean he, we could put him at like number one, right.
Speaker 1:I didn't stand his interview. That's awesome, that's that that warms my heart.
Speaker 2:Thank you, yeah, for that anecdote. Um, so uh, I'm gonna let you take this one away, but ai, uh, summarizing your chat logs and audio from seized mobile phones, what do you think?
Speaker 1:yeah, so there's this article, um, I don't know if you have you have the ur URL that can show people.
Speaker 2:I do.
Speaker 1:Yeah, so let's put it on. So 404 Media actually they're doing pretty good reporting on, I say, digital but cyber-y things, really good reporting. So they were mentioning and I want to make clear about this they mentioned how Celebrite is adding AI to their tooling and kind of going over their marketing materials and the claims they do and some possible issues with that. Now I want to make clear for everybody the article mentions Celebrite, but the fact of the matter is there's at least three or four tooling in the marketplace for digital forensics that have AI included, and when I mean AI, I mean generative AI, llms, because we had AI for other things in the past for image identification of guns of money. It's questionable how good or bad they are also to identify, possibly, csam images.
Speaker 1:So the technology exists which and from my perspective, no matter if it's LLM or not, it's pattern recognition. That's pretty much, I think, kind of like a baseline. You know, the keving at the bottom is pattern recognition. If that makes sense, llms themselves will sort of figure out what the next thing to say within the pattern of learned things, and of course they don't do that. It's an approximate calculation. You cannot calculate it fully because there's so many options. It would be impossible to calculate them all, so it does an approximation. That's why it's a model, right, it's not the thing, but something close to the thing. Like you have a car and you have a model car, it's the same car but smaller. Does that make sense? So the point I'm saying this is because these type of technologies are approximations to the ultimate calculations that cannot be made because they're impossible to be made.
Speaker 1:So, with that being said, I'm not picking on Celebrite, I'm not picking on any particular vendor. I will make some points that I found interesting about the article, and the main point is this All the promotional materials around marketing, and even us as users, right, always tells us that the tools, these AI tools, will find things that you can't find on your own and that they will do it faster. Right, they will find what you can find, and faster. That's the big promotional push from everybody. Actually, people themselves believe this to be the case, right, and I want to show a comment from Stacey Eldridge. Can you put that link?
Speaker 2:up.
Speaker 1:I will Yep Hold on one second so she's an excellent examiner and she used to work within the Bureau before she moved to the private sector, and she says that in her experience, ai is inconsistent. Right, you can do a task five times and the sixth one is all crazy and that's to be expected of these LLM technologies. That's nothing weird, that's just how it is right. You can look at an AI summary. She said summary, and you find nothing. You still have to go back. Right, but what if they miss something? So you end up asking about the chats but then having to read them and you find nothing. And I mean or and I'm gonna paraphrase her, just to not read it straight up but if you do find something, you still need to go back and and verify that's there, right, and read it, analyze, like she's saying right, what if the uh, if the uh ai has some bias, right, how will that influence the perspective? Right, and I agree with her. And I guess the point I'm making with this, with the article, is that, in regards to the marketing, when we talk about, this is a personal opinion doesn't reflect, and, as always, none of the things we say in the show reflect the opinions of our employers or anybody else. They, they just reflect our opinions and they're obviously subject to change as we learn new things. Right, we operate on principles, not beliefs.
Speaker 1:Now, that being said, the only way I think or opine that you will get a speed boost by using AI is if you make the habit of trusting the AI, of trusting the output. And the problem with trusting the output is that this output is a model, it's model-based. It has to add some random concepts to it to make it human sounding, and that's where the creativity of the thing that's needed to make it sound human will trip you up. It is okay to have AI gen AI modify your paragraphs on a whatever, on a speech you're going to give or a presentation. That's fine. But when we're talking about evidence, you can have that creativity there, right, and that will get you in trouble.
Speaker 1:So what happens? If you want the speed boost, you have to start trusting a system, not verify it, and the problem is that you cannot trust a system that you cannot validate, and it's a big difference. People talk about verification and validation. They're, and it's a big difference. People talk about verification and validation that are the same thing. You need enough verification points to validate a system, but you will never be able to validate a generative AI system. You can verify all the outputs, but if you verify the outputs, you're missing that speed gain right. So which one is it? Is it faster or not?
Speaker 1:I would argue it's going to take you at least twice as long Now looking at all of the data and going to verify it after the ai found it. Well, no, absolutely, I have you 100. And and I mean you have to be really careful, because we don't want to, we don't want to become, uh, a creator of questions. Does that make sense? Like, well, my skill is to create uh questions and the I tell me the answers. Um, like, look, at some point I need to do the thinking, I need to do the analysis. I can just offload that. I'm just gonna make questions and the ai gives me answers.
Speaker 1:Why, again, like stacy, saying what if it doesn't get all you need? What is if it's not the actual thing? Um, so, I guess you know, and some of the points that the article makes this is what Stacy said, but the article makes some good points. For example, this is an example of imagine this investigator and he's investigating people robbing stuff from porches right, like you know, like Amazon, packages that are being stolen from porches, and they pull this data there and the AI finds a pattern and then it becomes an investigation of a, you know, transnational ring of criminals stealing packages from porches. Well, hey, I would have never found that out without the AI. But the question is was your search warrant allowing you to do this Right? Do this right? Are you exceeding your authority in looking for patterns that you were not allowed to look for, because you came there for a specific purpose, not to see if there's a transnational ring of X or Y, right? And those are questions that are going to be played out down the road.
Speaker 1:I made a point in the threading LinkedIn of saying something we need to think about is adding disclaimers. The tooling is to add a disclaimer when output from AI is being pushed out to a report. There has to be some traceability of what is what the person does and what does the AI do, and if it's verified or yeah, verified or not. And I think we spend a lot of time thinking about what's good about it and not spending enough time thinking about what are the consequences of using this technology within the parameters that we have today, parameters that don't take into account AI, because that was not a thing three years ago and AI at this level right.
Speaker 2:Right.
Speaker 1:There is a lot of danger that we just be running through, and I'm talking about all the development in the field and also the users. We think people think AI is magic. Whatever you want AI to be, that's what it is. It's analysis, it gets unparsed data, it finds all the solutions and, actually, of the things that I said, ai does none of those. It does none of those. It will not find things that are unparsed for you. It will not give you all the solutions to a question, depending on how it's trained, what that model is.
Speaker 1:Imagine the article says that it's trained only on convictions. The LLM is only trained to get convictions. Well, there might be a data point where that person is innocent, but the data set, the model, is looking for convictions. And again, if you have enough data, you can find patterns in anything and it's going to find a conviction when there shouldn't be one. And you might think, oh, that will, I'll verify it, will you? Will you verify it? Are we sure about that? Are you sure that you're not? It's been right the last 10 times. It's surely right the 11th time, and I'm in a hurry. I got another 40 cases, 50 cases in the hopper that I need to work with right.
Speaker 1:The tool makers push it on the examiner and the reality is that the examiners being overloaded, they're going to. Many of them are going to assume it's right because the tools has it, because if it was wrong, why would they put on the tool? And that goes to the last point I wanted to make on this article reputational costs. Do you want to be the vendor that is brought out to court because the AI said some nonsense and you're going to stand? And you're going to be in the stand and say, whoa, we told the examiners to verify it. You can give that disclaimer on court, but the repetition of damage is going to be done. Your tooling will be known for spewing nonsense and your disclaimer is going to fall in deaf ears, right?
Speaker 1:So I believe there should be a more robust way of delineating what's AI, what's not AI of, either by policy or I don't know some technical solution to make sure that the examiner attests to the verification of AI-produced material, right, and again, first of all, because we want cases to run properly, like Brett's saying, the truth come out, but also, as part of the field and vendors, make sure that our tooling is providing what it needs to provide and our reputation will cost. Again, that's not even secondary, it's third theory. It's like a whole level down there in regards to justice. But look, if you apply a publicly traded company, for example, in this type of space, you need to worry about that. If you want the enterprises to continue to a publicly traded company, for example, in this type of space, you need to worry about that. If you want the enterprises to continue to subsist, does that make sense, heather?
Speaker 2:Yeah, according to this article too, like civil liberties experts are already latching on to the lack of transparency and inaccuracies in the AI generated results, so it's going to be questioned. It's definitely going to be questioned.
Speaker 1:Well, I mean, and you put AI in the tool and now becomes a really target rich environment for folks on whatever side of the investigative process is right, because it could be a civil dispute and one side is going to latch onto that right. The attack, the exposure right, the attack surface. When you put AI on the tool, it grows exponentially. On what things? What lawyers on whatever side could latch on to discredit the work? And so are we considering that? Are we considering how examiners will be exposed to argumentation because the tool has AI in it, right?
Speaker 1:Do we have I said it before something as simple as the prompts? Are the prompts being logged when they happen? What did they say? What the amount of responses that it got out of the responses, how many were correct, how many were incorrect? Do we have those statistics? I believe we don't, and I wouldn't be surprised that, again, attack surface has been broadened. Folks on the other side, with good reason, will ask for that right. How can we validate this process, right? Well, you can't. At a minimum, you have to have at least a traceability at a minimum, right, and I sound like a Luddite people that hate technology, but we don't. We love technology.
Speaker 2:Definitely.
Speaker 1:But there's some fields where we need to slow down a little bit. Right, this field is one medical. I don't want, I don't want AI giving me medicines.
Speaker 2:I was just going to say that. So you and I talk about AI so often that my Facebook feed is just filled with different AI models for different things, and most recently there's an AI specifically for lawyers and an AI specifically for the medical field. And I do not want my doctors diagnosing me off of AI. I see how he answers the questions I ask. If I, if I even ask chat GPT, uh, what does this artifact mean? They're always wrong, almost always wrong. I'll give it 90% wrong. And a doctor diagnosing somebody with that? We're going to have malpractice lawsuits coming our way, not our way, their way.
Speaker 1:The thing is about that I was asking. I didn't remember where our registry key was in Windows that I needed for a case and I'm like let me Google it right. But now Google on the top. They don't put the first search thing, they put the AI on the top. They do so you're forced to read that yes, and I'm like, well, let me go at it. And that registry doesn't exist. It's that registry that does not exist and I and I'm like what the heck?
Speaker 2:Those answers at the top of the page are wrong so often. I like to think that some of the maybe some of the tools I'm using, though, aren't as bad as ChatGPT, so hopefully we'll have some better results.
Speaker 1:but wow, and people don't understand how that works. You're going to start a sentence and the next part of that sentence, those tokens. Right, it could be a token, it could be a letter that's too hard to calculate but of word or phrases, that, based on the model, what should come next? Right, and then a little bit of random to make it sound human. And it's not really thinking what the right answer is, it's just thinking based on my model, the probabilities of my model, what would be the right thing to say or the closest thing to say, with a little bit of randomness to make it sound human. And that's scary for fields where you can not have an approximation to the truth. You need the actual truth. I need the actual medicine.
Speaker 1:I was reading an article where they were doing transcriptions from you know, the doctor records what you know. Today I met patient Alex Vignoni. I'm going to give him 200 milligrams of Pteradol, whatever. So that recording. There's people that actually go and type it out to get these prescriptions. Well, they have an AI do it, and AI was making mistakes in what the actual medication was or what the dosage was. People could die if they get the wrong medication, the wrong dosage, right, yeah, so I don't you know. We have to be taking that into account.
Speaker 1:I think the Utah Francis field, and not only the vendors but also us as users, temper our expectations. Learn about AI to make sure we understand what the attack surface is. And since the tools are going to have it, like it or not, because vendors already put them in, then you have to think as an examiner how will this new attack surface affect my cross-examination? When I'm being cross-examined, how will that affect how I write my reports? If I'm integrating AI knowledge, am I having the transparency that's required through the discovery by law, and can I substantiate my verification in the face of a tool that can never be validated? And those are things that we we start really think about yesterday, not today, yesterday.
Speaker 2:Yeah, definitely, definitely AI. I had to put up a couple of comments here. Ronan says it's. It is not me. Ai wrote this message. Hi, ronan, we know it's you. Thanks for joining us. And Forensic Wizard says it would make people lazy.
Speaker 1:And definitely, it's just easy to push the button and trust it I mean, I had a meme that says, uh, tooling enables mediocrity, uh, convince me otherwise, or something like that. I mean, anyway, it's not, it's. It's not. It's not in cheek, um, but the, the, the automation is the idea is to focus you on what you need to look into, but the way it's actually marketed is this is the solution to your backlog problem. Yeah, and they and you're off to the races and we've got to be careful.
Speaker 1:I think we should go back to the understanding that any tooling, ai or not, is to focus your attention on things that you need to take into consideration as you're doing your examination, but they're not the examination. The tool output is not the exam. The things that were highlighted by the tool is not the exam. You and me know this to be true. We look at a case and the most important things of the case are not parsed by the tool period and me asking AI about it. The AI will not know about it either, because it's not parsed. So I think we need to go back to those basics as examiners. This will focus my attention, but it's not the thing. It's not the. The data is the data. The reports are not the data. The AI outputs are not the data. The data is the data. The reports are not the data. The AI outputs are not the data. The data is the data. We need to go back to that.
Speaker 1:Yes, all right, so we're going to move on from the AI and folks. We said this a whole bunch of times. But guess what, as long as AI is being pushed down our throats, we're going to be sounding the alarm of we need to make sure that we take this seriously and do our responsibility, so you'll hear this in many more podcasts.
Speaker 2:I'm sorry to tell you Yep.
Speaker 1:But until AI changes in some fundamental ways, we're still going to be sounding that warning. So it is what it is.
Speaker 2:Agreed New blog post from Josh Hickman. The Binary Hick is his blog. He recently wrote a blog post about Apple CarPlay. It is his second blog post about this topic. He revisited the forensic analysis that he previously did on Apple CarPlay. The blog talks about how to distinguish between actions performed via CarPlay versus directly on an iOS. One of the artifacts this is just one of the artifacts he talks about. One of the artifacts this is just one of the artifacts he talks about are the unified logs and the wired and wireless CarPlay connections that you can find in the unified logs. He also outlines numerous other artifact sources that contain data related to the Apple CarPlay. This type of data, I would say, can definitely be used in your distracted driving cases. Was the driver on the phone? Were they manipulating the phone or were they connected to CarPlay and maybe going wireless? Might need to know that in a case like that. So his blog would definitely be helpful for anybody that is investigating a case that has those charges.
Speaker 1:Oh yeah, there's a pretty common cases that require that, so please check that out.
Speaker 2:Ah, let's see here. I'm reading comments and switching in here, so hello, who is on the line is another blog post that recently came out by Metadata Perspective. It looks into forensic analysis of iOS devices to identify participants in group calls group calls I'm sure everybody here who does digital forensics has seen that maybe their forensic tools don't necessarily parse all of the participants in a group call. I think they've gotten better, but you used to not get all of the participants in group calls. So this investigates the call history store data database and focuses on the call record, the Z handle and the remote participant handles tables. Talks about how examining these tables and employing specific SQLite queries can help you uncover the details of a group call and its participants.
Speaker 1:And this is something they need to take into account, not only for this type of data right, but for any chatting application, and I mentioned this before.
Speaker 1:Some co-workers from another division found that when they look at a particular application chatting application and they look at the users that were participating in that group chat, the database had a field that explained not explained but showed if the person that's participating was an administrator of the channel or not.
Speaker 1:And that became really important because there's some federal law that states that if you're doing certain crimes online, if you're the administrator of the group that's making those crimes, you get a higher penalty for being a leader, right? And how do I prove that? Well, by showing you're an administrator, because you have the power to, you know, add people to the crime group, take them out. You have the power to even to stop it as an administrator, but you don't, right. So there's a lot of responsibility for administrators, and the tooling at the time did not show that data. It just showed the users and the conversation, but it didn't show if they were administrators or not. And the only way to find out is by you, the examiner, to looking at the source data and see what it says, what it shows.
Speaker 2:And utilize the blogs that are out there. This blog will show you the queries that you need to pull that data out of the database and also tells you what tables you need to look at. Don't always have to go and recreate the test data yourself. It may be out there already absolutely all right, so we're trying a new thing. Um, we have the what's up with the leaps, we have the meme of the week and now we're gonna have an artifact of the week.
Speaker 2:Um, yeah, so we'll do that as a new addition and we'll highlight an artifact that may be helpful in people's cases. So this week specifically, I'm going to show everybody kind of like the lifespan of a file that gets deleted from the gallery on an Android device. So let me share my screen here. So the gallery trash on an Android device, Starting in Android version 9, if an image or video is deleted, the file is renamed and sent to the following path Data media, zero, Android data, the ComSec Android gallery, 3D Files, and then trash.
Speaker 2:So that file will remain in the trash for 30 days. During those 30 days the user can go into the trash and permanently delete the file or restore the file to wherever its previous path was, which could be the DCIM, the camera folder. But if it's not restored within those 30 days, it'll automatically be deleted from that trash. So I have up on the screen just some screenshots that show what the trash on my Samsung device looks like, and then if I go into that trash bin, I have some files in there and at the bottom of each file it tells how many days left that file has in the Samsung's trash bin.
Speaker 1:I think you mentioned that with Pixel's phones is the same thing.
Speaker 2:Yeah, the screenshot I show at the end will actually be from my Pixel. Excellent, this is just a couple of screenshots from parsed files. I used Celebrite for this. A couple of screenshots from parsed files. I used Celebrite for this, and these are parsed files that are in that trash directory. You can see the file name. That's what it gets renamed once it's in that trash directory. So if you're looking at parsed data, you're going to see that new file name. You're going to see created dates, last access time, which are usually related to that trash bin, not necessarily when the file was created, and then, if you're lucky, you'll have some exit data there as well.
Speaker 1:And that file name seems to be like a big integer with a minus sign on the front. So people that are listening, they have an idea of what the file name entails it's a big integer number, really large, with a minus, kind of like a minus sign on the front.
Speaker 2:Some of them have the minus and some of them do not have the minus, and I haven't figured out exactly why yet. But we'll work on it and when I do we'll share.
Speaker 1:Absolutely.
Speaker 2:Let me just I'm going to remove this from the screen and share a screenshot that I have from my pixel. So the screenshot I have on the screen now shows the database that you'll find information about the files that reside in that trash. You will find it's called local DB. Let me zoom in so everybody who's watching can see. So the database is local DB. Oops, hold on, I'm on the wrong screen. Zooming in, that doesn't help. There we go. So local DB. And if you look at the tables in the local DB database you see the trash.
Speaker 2:I have four files in my trash on my pixel device. In the ABS path column you'll see the path that they're currently residing in, which is the trash, and that long integer number that the file has been renamed to when it was deleted. Scrolling over just a little bit, there's origin path, which will give you the original path and file name related to the file that has been deleted. So all four of my images were originally in the DCIM camera folder and they were named 2025-02-27, like the normal naming convention that you'll see for the Samsung device. So all four of these images were taken with the Samsung device. I know that because I took the pictures.
Speaker 2:And then another column of interest is the delete time. So all of these images were deleted on 227 at 206.56, and you can find that right in the delete time column. Further over there's a column called restore extras and I have that restore extras up on the end column here and it has additional information about the file. So this is an image file. If it had been a video file, the duration is in here, the original path is in here, but the date taken is also in this restore extras column. The date taken can be brought over to a tool like decode to be decoded, and you'll see that this picture in particular was taken on 2-27 at 2-06. And if I come back up, it was deleted at 2-06-56. So just seconds from when the picture was taken it was deleted and sent to that trash where it will remain for the 30 days unless I restore it or let that 30 days expire and it removes permanently.
Speaker 1:That's, that's fantastic. You know, can you imagine? It's like this picture was taken whatever weeks ago and then it got deleted, and right before, right right after we knock on the door.
Speaker 2:Yeah, so I've had that happen, it's not. There's a couple of cases I've had that happen and and you won't know this you won't know that they were all deleted the morning that the officers were knocking on the door. You won't know what its original name was or where its original path was, or if it was potentially taken with the camera, if it's in the DCIM camera, unless you go into the database and do some manual analysis yourself, unless you use ALEAP. Because as soon as I found this, I called Alex. I didn't know how to do the ALEAP, ileap stuff. It was quite a few years ago and I said can you support this in Elite please?
Speaker 1:so I'll have a pretty report for my case. And he did. Yeah, we try to please.
Speaker 2:We aim to please. Ah, I've got messages in here that say we can't see it. Did everybody see the screenshot?
Speaker 1:I don't know. I mean, I'm seeing it. Let me, let me go to the uh, let me go to the uh live channel, let me see if it's what's the deal.
Speaker 2:My sister wrote Damn you AI.
Speaker 1:Don't change it. Oh, that's true, it's not showing. Oh no, it's just a blank space. I went to the channel and see, just to see.
Speaker 2:Okay.
Speaker 1:Yeah, let me see what the deal is.
Speaker 2:I'm going to remove it from the screen and re-add it. No, no, no.
Speaker 1:Leave it up there, leave it up.
Speaker 2:No, no, no, no, leave it up there, leave it up.
Speaker 1:All right, I leave it there, and what else?
Speaker 2:I'm totally going to share it out on the on the on the podcast page. I apologize, I've just explained it all. Well, it's like you're listening to the audio podcast without the YouTube version, with the video right.
Speaker 1:Yeah, no, for sure, for real. I'm looking, I'm spying on YouTube and it's like it's a blank space. That's just so odd. So uh, yeah, you know, I mean.
Speaker 2:I will share the screenshot. So I took, um, I took and drew boxes around, uh each of the columns that I just mentioned and I uh put depth, uh not definitions, but uh explanations of what the data is in each of those columns. So I will share that out with everybody.
Speaker 1:Yeah, we will. I'm sorry. I'm just kind of testing out if I make it full screen, if it shows, but I don't think that's going to happen. Yeah, I made it full screen. I can see it. Can people confirm they're seeing it when I put it full screen, because I think I'm seeing it.
Speaker 2:Holly, can you see that?
Speaker 1:I know my sister's listening, yeah yeah, they're seeing it, you know what it shows. Now, yeah, I make it full screen. It seems that that's that sizing. It makes it messes it up, but I mean, let's, can you just like, like quickly, kind of, since now they can see it, can you quickly talk about the colors, like this box has this oh yeah, colors. And no, don't zoom it in, just leave it there, at least they can see it.
Speaker 2:So so the green box is in the column labeled ABS path and that contains the path that the file is currently in in the trash with that long integer name. The red box is the original path and file name related to that trashed file. Name related to that trashed file so it will be for the for my files is DCIM camera and then the original file name of the images that I took with this device's camera. The blue box is the delete time. All four of these images were deleted at the same date and time, so I have the same date and time for all four. And then over on the left hand side is that data that I said was in the column called Restore Extras and it includes the date taken which I have in a purple box, and down on the bottom is another purple box in the decode tool showing the conversion of that timestamp.
Speaker 1:Excellent. So at least people got a, the ones that are live get a quick or watching the recording later can see what the database kind of looks like and the decoding process to get that timestamp so pretty good stuff.
Speaker 2:I just went on gabbing and didn't even check the comments.
Speaker 1:I don't know. It happens and again, there's a lot of changes on our system that we use for for the podcast. So that might be one of the issues. We had to email the developers and say, hey, this might be happening, and then they can fix it. I'm going to take it out of, I'm going to remove it, okay, and then whenever we show the I took ourselves out, whenever we show the meme of the week, then oh, hold on, I don't like this, hold on, I like to be on the next. Ah, that's better.
Speaker 1:Yeah, see, I get antsy, all right. So whenever we show the meme of the week, let's make it full screen, so hopefully that will show. Oh, yeah, definitely.
Speaker 2:And I'm going to share out that picture for the deleted gallery. I'm going to put it right on my LinkedIn and on the podcast LinkedIn so everybody can see that.
Speaker 1:Excellent.
Speaker 2:All right. So we are to what's new with the leaps. I know that there is a ton of stuff new with the leaps. There's all kinds of stuff going on in the background by a bunch of amazing people. I'm just going to highlight one update to iLeap this week. So there was an update to the health parsers, kevin Pagano, who is in the chat. He added location type to location type for activity, so indoor and outdoor. For the health parsers on iOS, he had a new parser for source devices, which includes make model software and other other devices and other devices. So if you're looking at the health parsers in iOS, you can go in now and see if activities were indoor versus outdoor as logged in that health database.
Speaker 1:Well, and that's no, no, and that two things. The first is that really stresses the point that you might be used to or really know really well a data source. Oh, I've done this data source 20 times and it's always you know. Depending on the case, give it a do-over real quick because you can find new stuff in old databases. There's nothing that prohibits the vendor that's making the tool or the product, I should say, to add a new table with useful stuff. And Kevin is just finding more stuff on a database that we thought we knew all about, but there's always something new that could come out. So that's pretty good.
Speaker 1:I want to really give a quick shout out to Johan Polacek. He's the best. He's been doing a lot of work underneath the hood of the leaps, specifically to make it for Lava and those that are not aware. It's a new system that we're developing to be able to deal with large data sets and be able to present it to you, no matter how large that data set is. So because currently the Leap tooling is HTML-based reporting and HTML-based reporting breaks really easily if it deals with a large data set like a large report. So we're working on that.
Speaker 1:Johan is actually working on the media section to show media within Lava from the Leap side, and he's doing an amazing job of that. I'm trying to get a hold of my work situation and wait till things settle down a little bit and then I can hopefully then add to that effort migrating artifacts to the new Lava system and helping with other general coding responsibilities. So I know I've been a little bit out of action, but the hope is that soon enough we can start working on that. So, again, thanks to Kevin.
Speaker 2:Kevin just even saying that there's more Apple health coming up, so that's pretty exciting yeah definitely, and we are at the end of the show to the meme of the week, so let me share my screen let me confirm that we can see.
Speaker 1:Let me spy on the yeah, can we? Let me spy, so let me see, let me see. Okay, we can see it so it was just that one screenshot. We can see it, I can see it, so it was just that one screenshot. Hmm, weird, yeah, I can see it, I can see it.
Speaker 2:The meme of the week goes along with our AI chat. This week we have an iceberg on the meme and above water, what the AI finds, and then below water, what only you can find.
Speaker 1:Well, and the topic with icebergs is that. You know, it's the tip of the iceberg, right?
Speaker 1:Yes, it is Usually the tip is the smallest part of the iceberg. What's underneath is what can sink the Titanic. Right, right, exactly. And that's me trying to give a visual exhortation to examiners to be aware that, yes, we have backlogs, yes, we have a lot of cases, yes, we're overworked Right now, budgets are being cut, people are being fired and we have to do more with less and less and less, and I get that.
Speaker 1:But that just means that we need to be more clear-eyed in what's important. And how do we get there and not think that, if we offload the responsibility to a system, that that will take care of the issue? Most likely it will not. So we have to think about and it could be a topic for a full episode how can we be more efficient, how can we speed up our verification, validation procedures and how do we do a workflow that maximizes the identification of key items in a case in order to be able to be as efficient as we can with what we have? But again, it's just a way for me to really motivate people to or at least have that into account.
Speaker 2:Absolutely All right. That's all I've got, that's all we got.
Speaker 1:Yay, I mean. Again, thank you everybody for for sticking with with us. Um, again, there's a lot of things going on around the world things going on at work, things going on in our personal lives, even car crashes going on that's enough of that, yeah please no more.
Speaker 1:Um. So again, life is life and and we all live it together. So we appreciate your understanding and we'll try to be as consistent as we can and hopefully, you know, things get better for everybody and we'll keep trucking here and giving you the latest on data forensics and our opinions on those things. Yeah, anything else for the good of the order, heather.
Speaker 2:That's it. That's all I've got.
Speaker 1:All right, well, everybody, we'll see you all soonish again. Thank you, everybody.
Speaker 2:Those are live the ones that are watching.
Speaker 1:Thank you for that, those hearts, we love you as well and I will see you on the on the next episode. Yeah, thank you. Have a good night bye, thank you. Outro Music.